Current large deep learning models improve accuracy through complex network structures but sacrifice runtime speed and increase the number of parameters. To address this issue, we adopted the “pruning” technique, which makes deep learning models more lightweight and efficient.
Pruning is like trimming a tree, removing unnecessary parts to simplify the model structure, thereby improving inference speed and reducing memory usage. This technique not only enhances the model’s efficiency but also reduces resource consumption, making it suitable for deploying on hardware platform with limited computational resource.
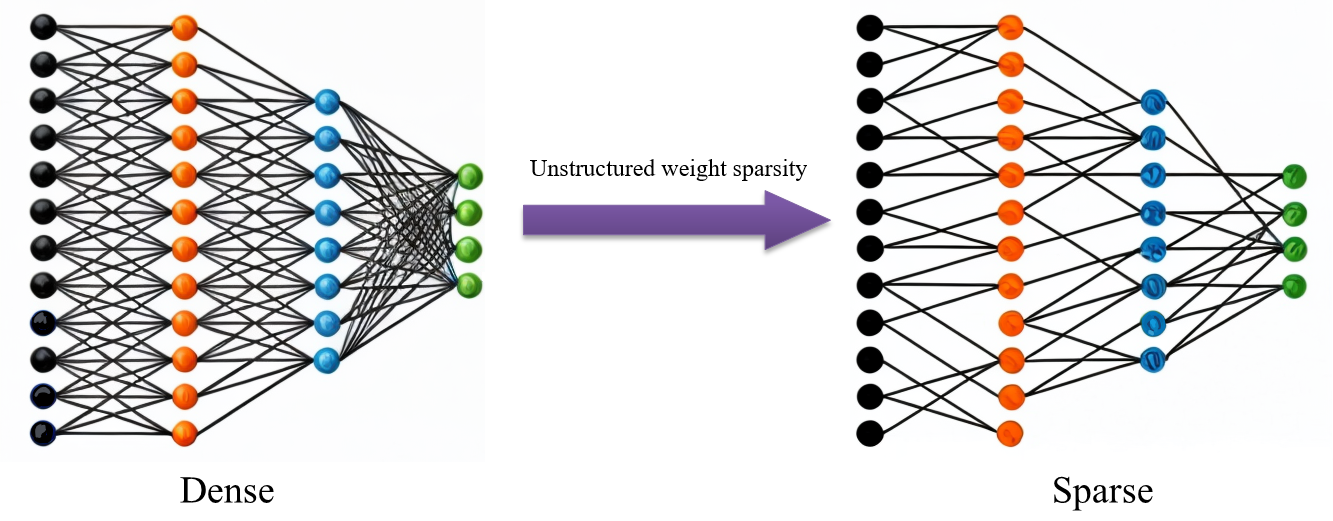
Our technology includes:
Sparse Training: By enforcing sparsity during the training process, we gradually reduce unnecessary parameters, resulting in a smaller and more efficient model. Sparse training can significantly reduce computational cost and memory usage while maintaining the model’s performanceat the same time.
Structured Pruning: This technique removes entire neurons or convolutional filters so as to reduce the complexity of the model. Structured pruning ensures that the pruned model maintains a regular structure, which helps optimization of hardware accelerators and deep learning frameworks.
Fine-Tuning After Pruning: After pruning, the model was fine-tuned to recover any performance decay caused by pruning, ensuring that the model’s accuracy and stability are maintained.

By applying these techniques in combination, we can drastically reduce the resource requirements of the model while preserving its performance, offering an effective solution for model deployment on real-world platforms.
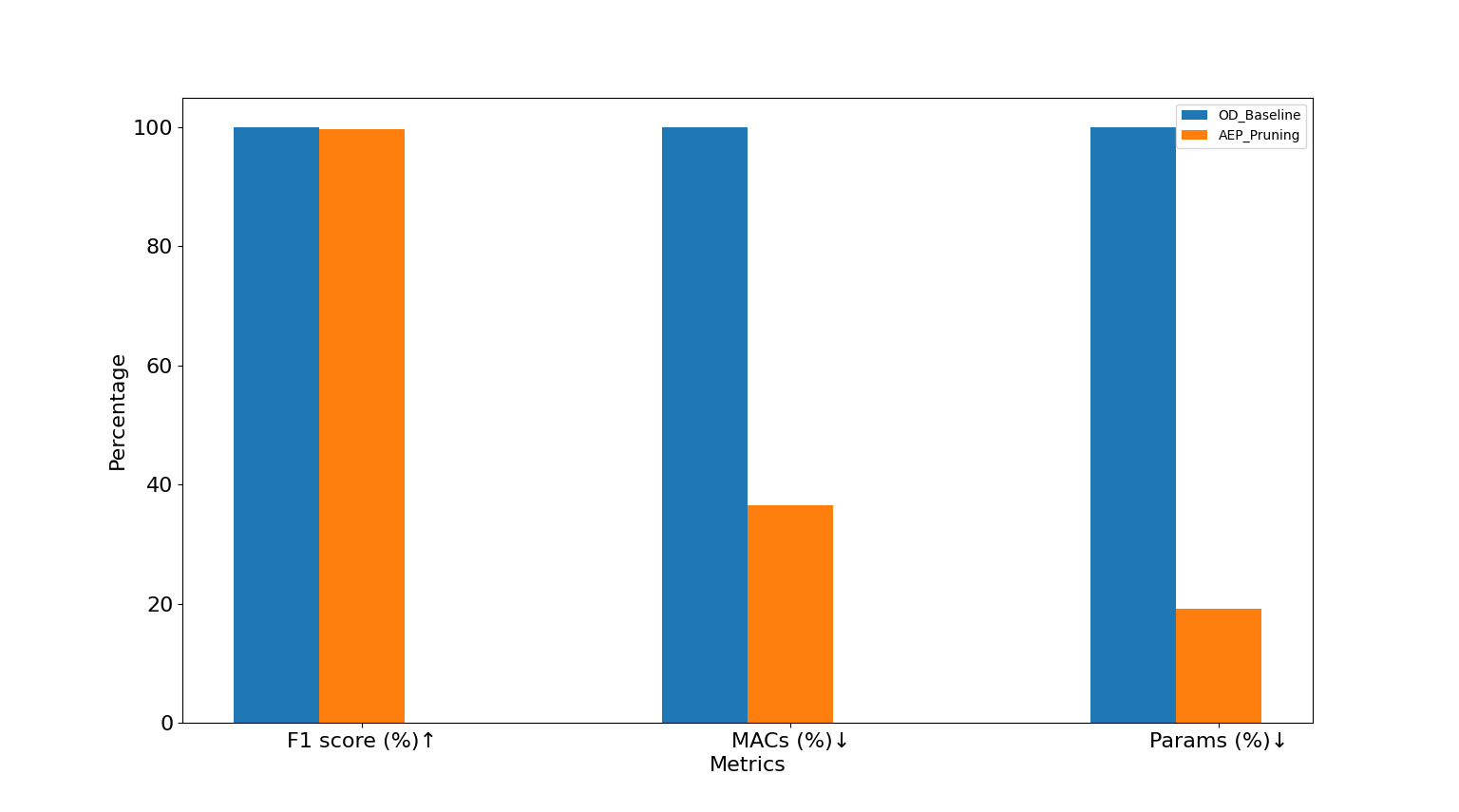