As AI technology becomes more and more mature andapplied to many fields, there is increasing concern about how AI makes decisions, especially in rigorous domains.
In the automotive electronics industry, which is a strict field. When applying AI to automotive field, it is important that the AI's decision-making process is reasonable.Besides verifying the accuracy of AI through data validation, model explanation methods are also needed to ensure that AI learned the correct features. Therefore, we developed a method for model explanation to check our object detection model if it has learned the correct features.
We also utilized model explanation technology for the following applications:
1. Based on the characteristics of the model and the model explanation methods, we have established metrics to evaluate whether the model has learned reasonable features. This helps in adjusting the data collection direction, as more complex scenarios for the model can be addressed by increasing the data to improve model learning.
2. We use model explanation technology to compute similarity between different categories so as to check if model confuses the objects with high similarity.
3. We simulate real-world scenarios where the camera may slightly change its mouting position and angle, and then we use model explanation technology to observe the features of model learning. This helps to verify the robustness of the AI model and ensures that the model can still maintain accuracy in practical applications.
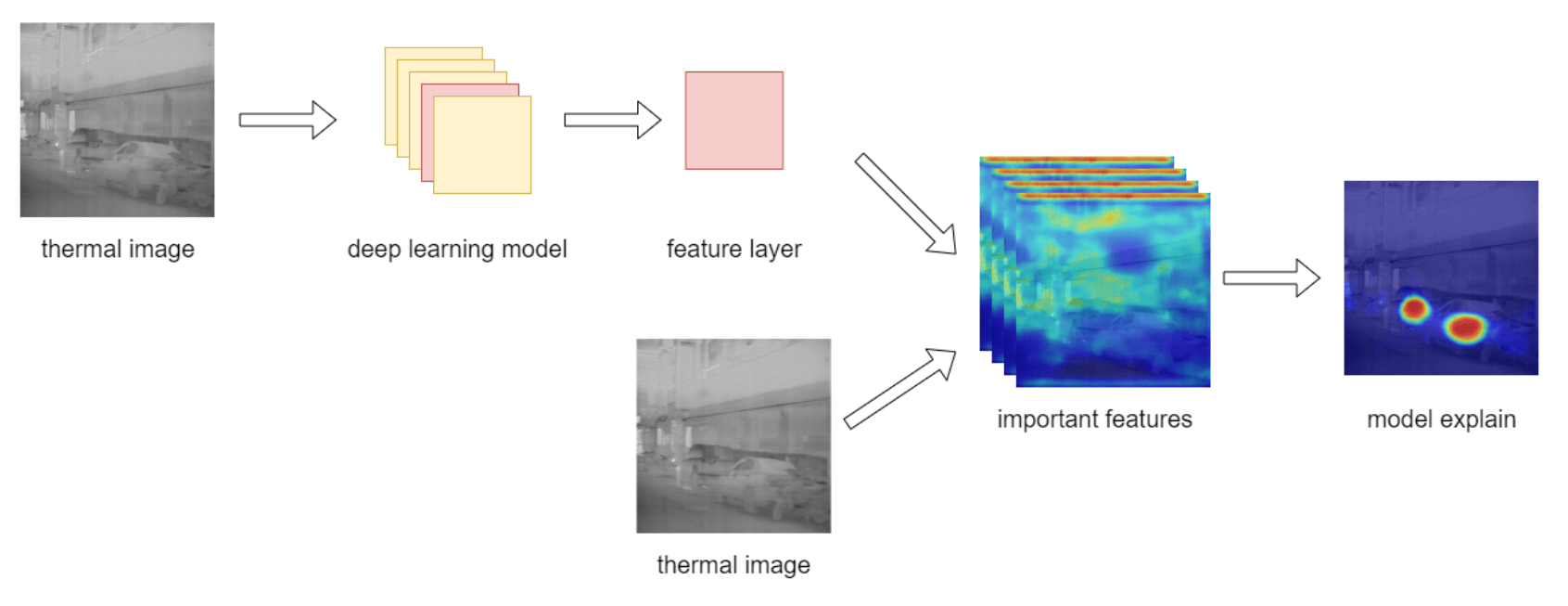
Result