Training AI models requires a large amount of human resources to gather sufficient data. To help AI models learning real-world scenarios, we need to have diverse datasets. However, in fact, many types of data are difficult to obtain. Therefore, traditional image augmentation techniques are often used to increase data volume, but these methods have limitations when it comes to meet diversity requirements.
In order to solve this problem, we developed generative AI to replace traditional image augmentation techniques. Generative AI can create more diverse and realistic images, which not only improves the quality of the dataset but also enables the AI model to learn various real-world scenarios. Through generative AI , that allows us to more efficiently obtain the necessary data while reducing human resource.
Method
Modern generative AI includes several different types, such as Autoencoders (AE), Variational Autoencoders (VAE), Generative Adversarial Networks (GAN), Normalizing Flow, and Diffusion Models.
These models can be trained for tasks like noise-to-image, text-to-image, and image-to-image generation, depending on the product needs and available resources.
For our product, we chose the image-to-image training approach. By using paired datasets to train the model, we can precisely control the outline of the generated images at the input side and use the model for style transformations, such as changing weather conditions or scenes. This method not only enhances the quality of the generated images but also increases the model's flexibility and range of applications, meeting diverse product requirements.
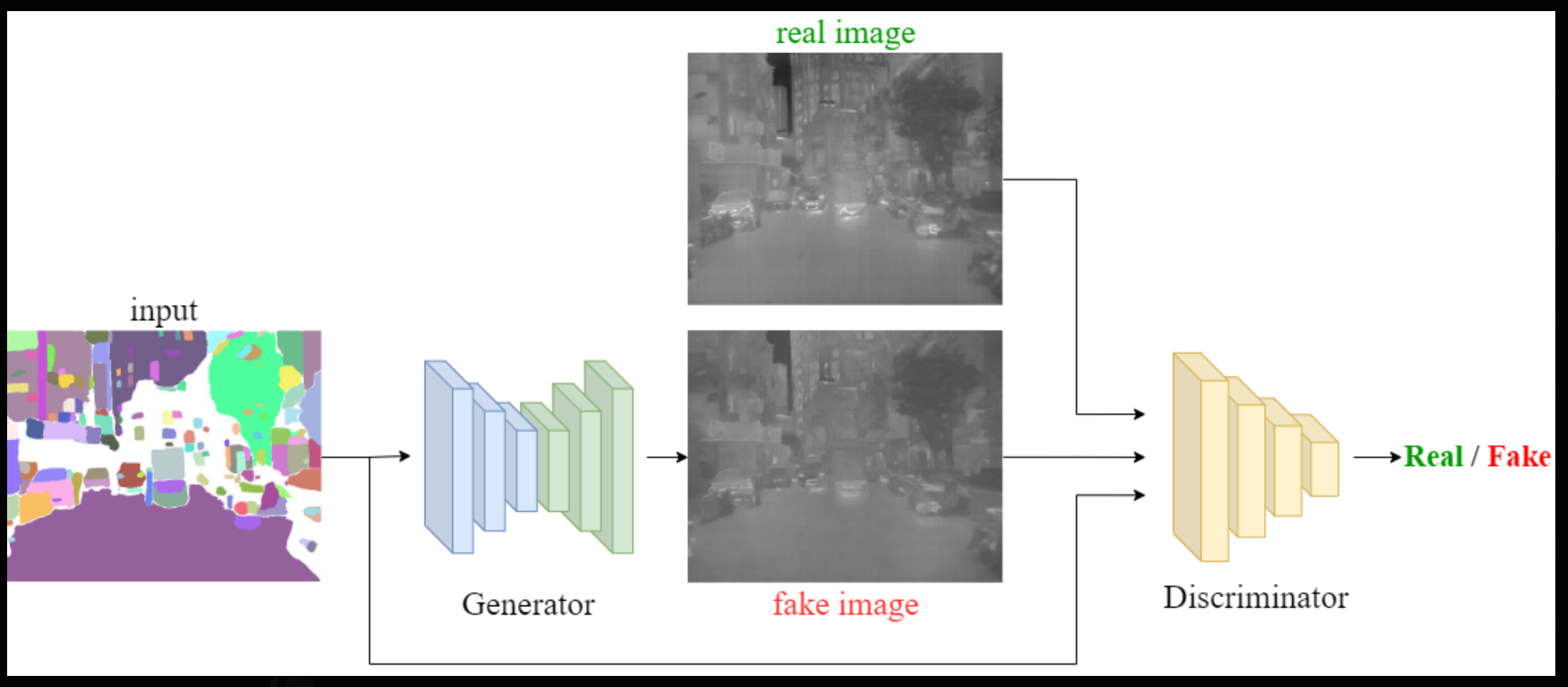
Result
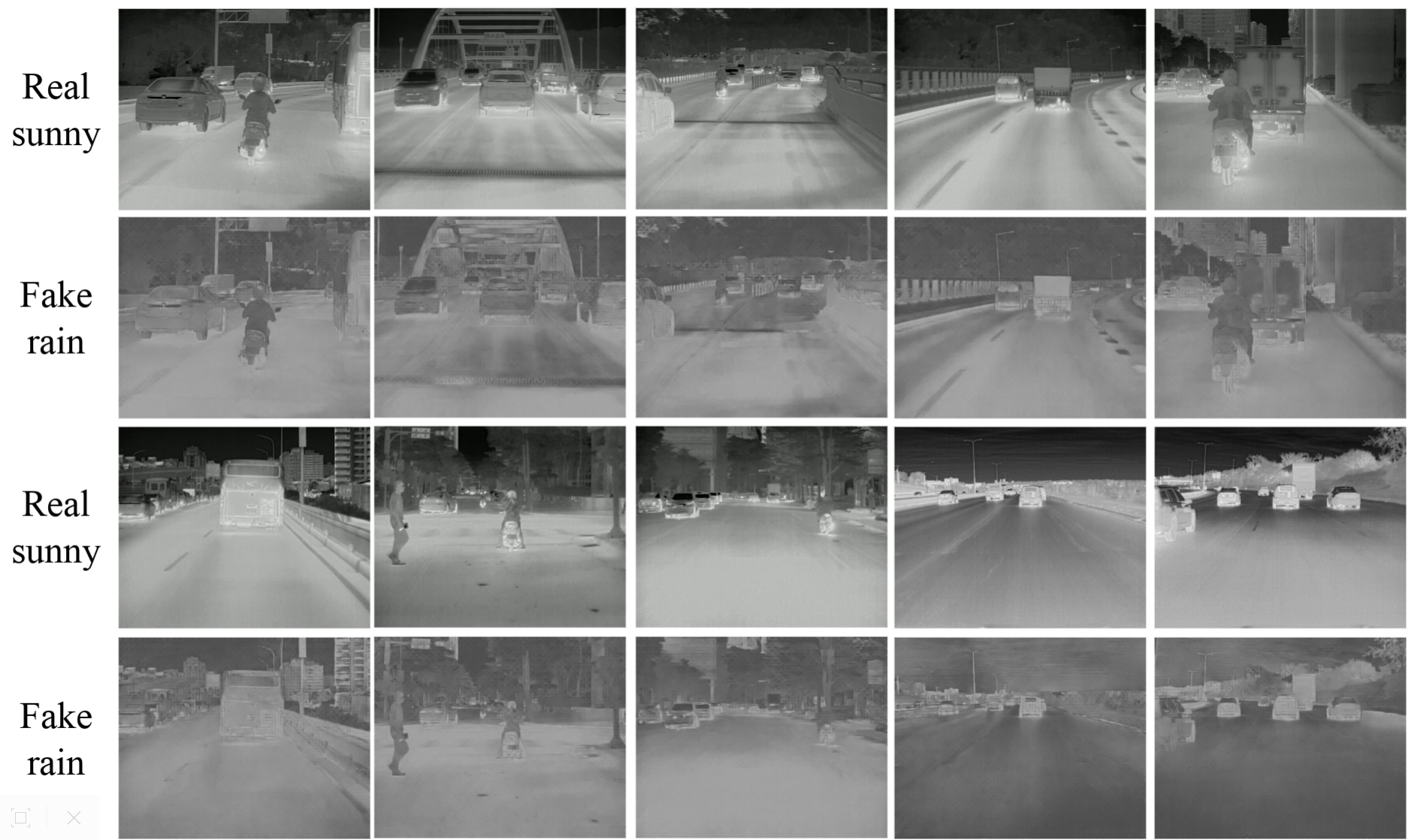